In order to correct the real life bias and barriers that prevent women, girls and all people marginalized from achieving full participation and rights in the present & the future we invent, we must ensure that machine learning does not embed already biased systems into our collective futures.
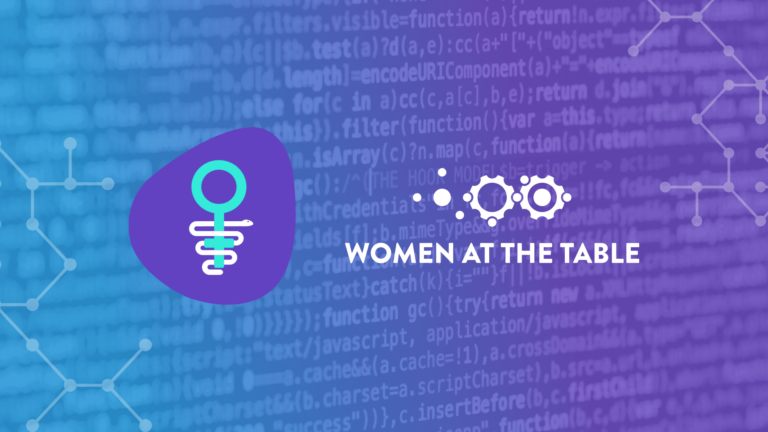
The Gender Data Health Gap: Harnessing AI’s Transformative Power to Bridge the Gender Health Data Divide
The gender data gap is turbocharged by AI.
In the context of continuing and widespread AI adoption in healthcare, we run the serious risk of structurally embedding biases and gaps. Without being aware. Again.
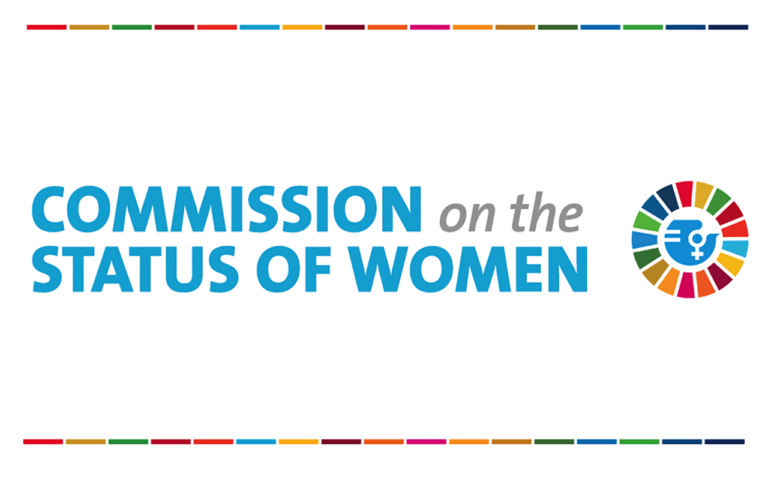
Artificial Intelligence to Advance Gender Equality: Challenges and Opportunities
Interactive Dialogue on the Emerging Issue – CSW68, 22 March 2024
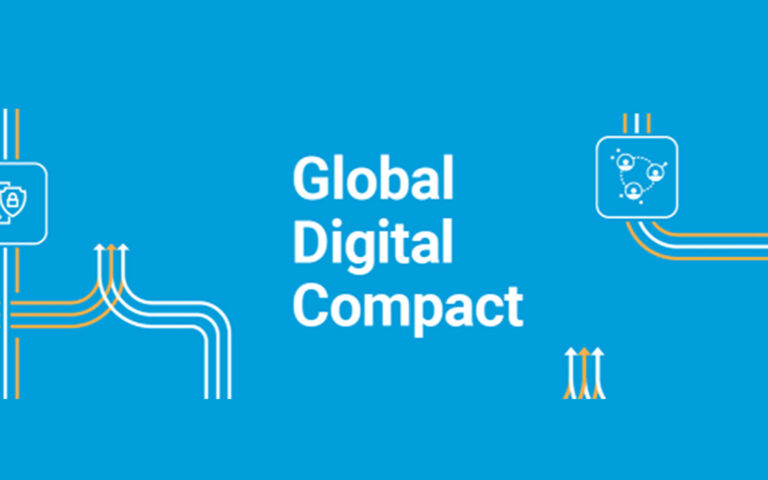
Gender at Heart of the Global Digital Compact
Event hosted by Finland and the Action Coalition for Technology & Innovation for Gender Equality.
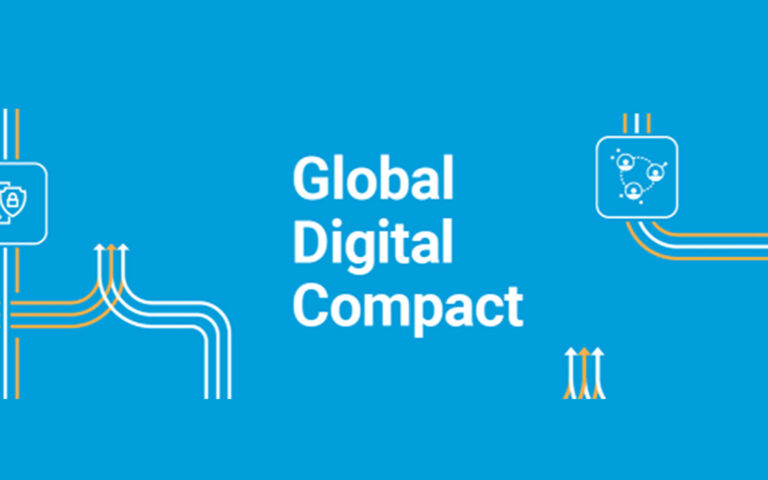
Algorithmic Accountability as Human Right
This is our oral statement to the Global Digital Compactco-facilitators, Rwanda and Sweden, outlining our vision for algorithmic accountability as a human right.
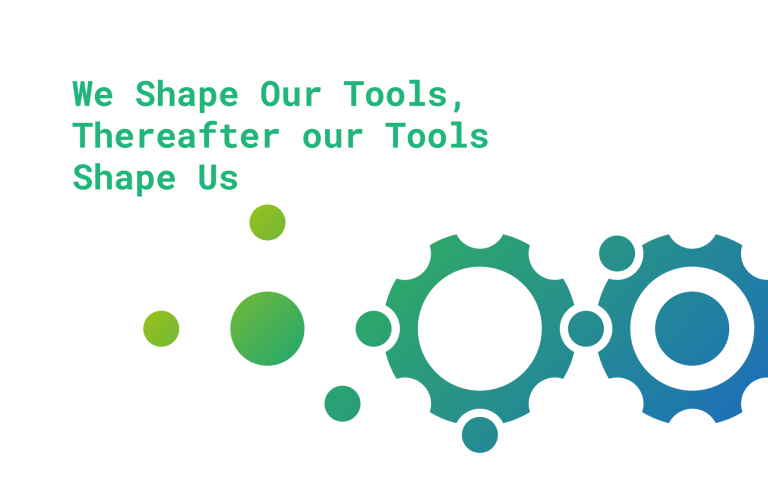
We Shape Our Tools, Thereafter our Tools Shape Us
Our foundational paper from 2019 harking back to media critic Marshall McLuhan’s statement that “We shape our tools and thereafter our tools shape us” giving a full landscape and set of recommendations on ways to invent a more equitable future with the algorithmic tools we create.
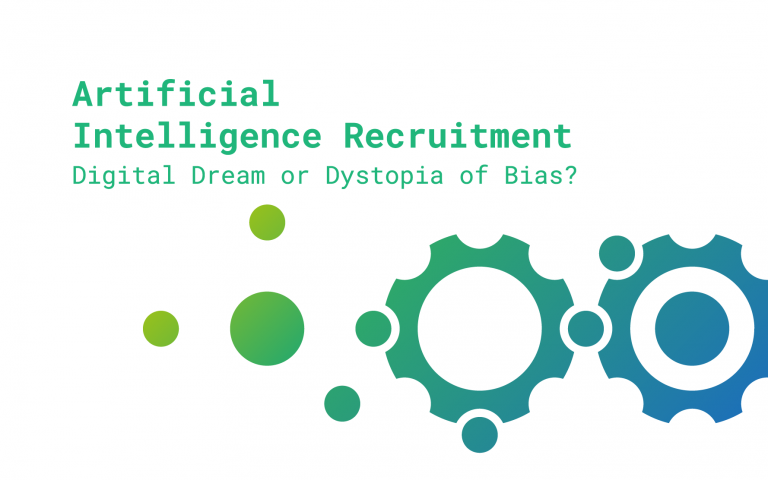
Artificial Intelligence Recruitment: Digital Dream or Dystopia of Bias?
In a Post-COVID world we turn more and more to online recruitment. How does this effect those already left out of the system and the data. This paper written in collaboration with a team from Skadden,Arps looks at three jurisdictions, UK, EU/ France, and the US to further understand what is already happening and where the law might go.
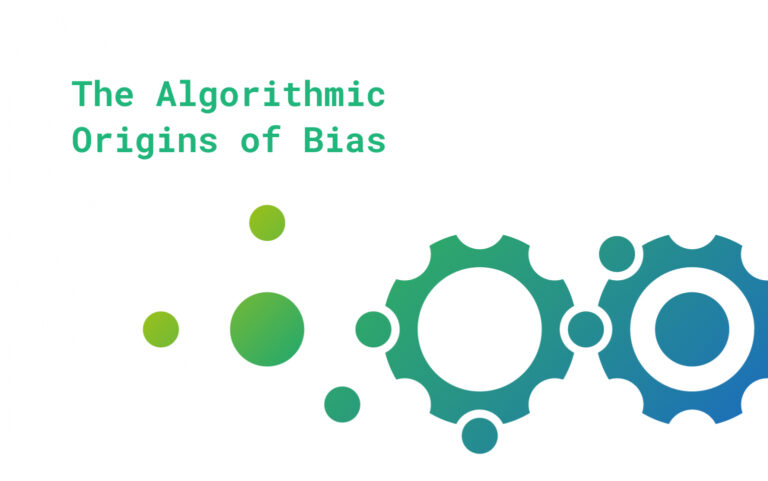
The Algorithmic Origins of Bias
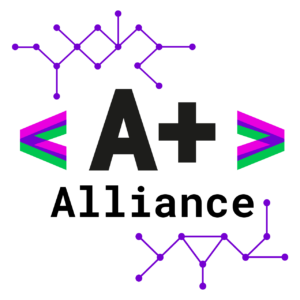
< A+ > Declaration
Written for a Keynote at Women in Data Science Zürich 2019, this Call to Action has become the manifesto for the <A+> Alliance and all of its, and Women at the Table’s work.